Big Data Analytics Architecture: Unlocking the Power of Data
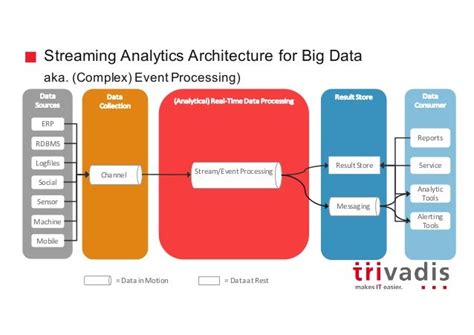
Big data analytics has emerged as a game-changer in today's data-driven world. With the exponential growth of data, organizations are realizing the need to harness the power of big data to gain valuable insights and make informed decisions. However, analyzing massive volumes of data requires a robust architecture that can handle the velocity, variety, and volume of data. This article explores the intricacies of big data analytics architecture and its role in unlocking the power of data.
Understanding Big Data Analytics Architecture
Big data analytics architecture refers to the framework and infrastructure required to process, store, and analyze large volumes of data. It encompasses various components, including data sources, data storage, data processing, analytics tools, and visualization. The architecture is designed to handle the three V's of big data: volume, velocity, and variety.
Components of Big Data Analytics Architecture
1. Data Sources:
The first component of big data analytics architecture is data sources. These can include structured data from databases, unstructured data from social media, sensor data, log files, and more. Data sources can be both internal and external to the organization.
2. Data Ingestion:
Once the data sources are identified, the next step is to ingest the data into the analytics system. Data ingestion involves collecting, cleaning, and transforming the data into a format suitable for analysis. This process ensures that the data is accurate, consistent, and ready for further processing.
3. Data Storage:
After data ingestion, the data needs to be stored in a scalable and efficient manner. Traditional relational databases may not be suitable for big data analytics due to their limitations in handling large volumes of data. Instead, organizations often opt for distributed file systems like Hadoop Distributed File System (HDFS) or cloud-based storage solutions like Amazon S3.
4. Data Processing:
Data processing is a crucial step in big data analytics architecture. It involves transforming and analyzing the data to extract meaningful insights. This can be done using various techniques such as batch processing, real-time processing, or a combination of both. Technologies like Apache Spark, Apache Flink, and Apache Storm are commonly used for data processing.
5. Analytics Tools:
Analytics tools play a vital role in big data analytics architecture. These tools enable data scientists and analysts to explore, visualize, and analyze the data. Popular analytics tools include Apache Hadoop, Apache Hive, Apache Pig, and Apache Mahout. Additionally, machine learning algorithms and artificial intelligence techniques can be applied to uncover patterns and make predictions.
6. Data Visualization:
Data visualization is the process of presenting data in a visual format, such as charts, graphs, or maps. It helps in understanding complex data and communicating insights effectively. Tools like Tableau, Power BI, and D3.js are commonly used for data visualization in big data analytics.
Challenges in Big Data Analytics Architecture
While big data analytics architecture offers immense potential, it also comes with its own set of challenges. Some of the key challenges include:
1. Scalability:
As the volume of data continues to grow, scalability becomes a major concern. The architecture should be able to handle increasing data volumes without compromising performance.
2. Data Integration:
Integrating data from various sources can be complex, especially when dealing with different data formats and structures. The architecture should provide mechanisms to integrate and harmonize diverse data sources.
3. Data Security:
With the increasing importance of data privacy and security, the architecture should incorporate robust security measures to protect sensitive data from unauthorized access or breaches.
4. Real-time Analytics:
Real-time analytics requires processing and analyzing data as it is generated. The architecture should support real-time processing capabilities to enable timely decision-making.
5. Cost Management:
Implementing and maintaining a big data analytics architecture can be expensive. Organizations need to carefully manage costs associated with infrastructure, storage, and analytics tools.
Benefits of Big Data Analytics Architecture
Despite the challenges, big data analytics architecture offers several benefits:
1. Data-Driven Decision Making:
By leveraging big data analytics, organizations can make data-driven decisions based on insights derived from large volumes of data. This leads to improved operational efficiency and better business outcomes.
2. Improved Customer Experience:
Big data analytics enables organizations to gain a deeper understanding of customer behavior and preferences. This allows them to personalize products, services, and marketing campaigns, resulting in an enhanced customer experience.
3. Competitive Advantage:
Organizations that effectively leverage big data analytics gain a competitive edge by identifying market trends, predicting customer needs, and staying ahead of the competition.
4. Innovation and New Revenue Streams:
Big data analytics can uncover hidden patterns and insights that drive innovation and open up new revenue streams. By identifying untapped opportunities, organizations can develop new products, services, and business models.
Conclusion
Big data analytics architecture is the backbone of any successful big data analytics initiative. It provides the foundation for processing, storing, and analyzing large volumes of data to extract valuable insights. By understanding the components, challenges, and benefits of big data analytics architecture, organizations can unlock the power of data and gain a competitive advantage in today's data-driven world.
Learn More :
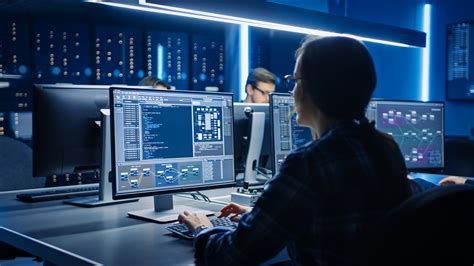
In today's digital age, data has become the lifeblood of businesses across industries. The ability to collect, analyze, and derive insights from large volumes of data has become crucial for organizati...
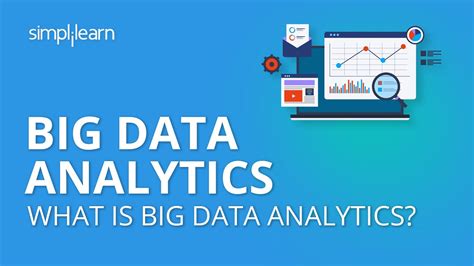
Big data analytics refers to the process of examining large and complex datasets to uncover hidden patterns, correlations, and other valuable insights. It involves the use of advanced analytics techni...
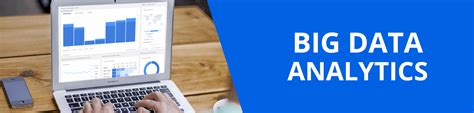
In today's digital age, data is being generated at an unprecedented rate. From social media posts to online transactions, every interaction leaves a digital footprint. This massive amount of data, kno...
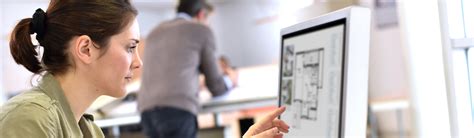
Big data analytics has emerged as a game-changer in various industries, revolutionizing the way businesses operate and make decisions. With the exponential growth of data in today's digital world, org...

In today's digital age, businesses are generating and collecting vast amounts of data from various sources. This data, often referred to as big data, holds immense potential for businesses to gain val...
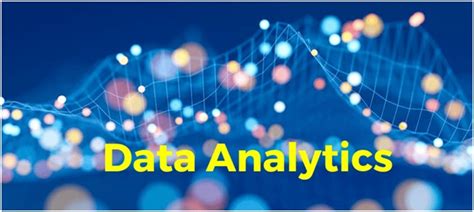
Big data analytics refers to the process of examining large and complex data sets to uncover patterns, correlations, and insights that can be used to make informed business decisions. With the increas...
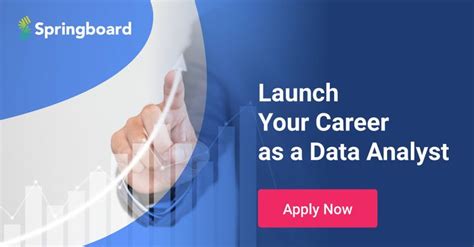
In today's digital age, data has become one of the most valuable assets for businesses. With the increasing amount of information being generated every second, companies are now turning to big data an...
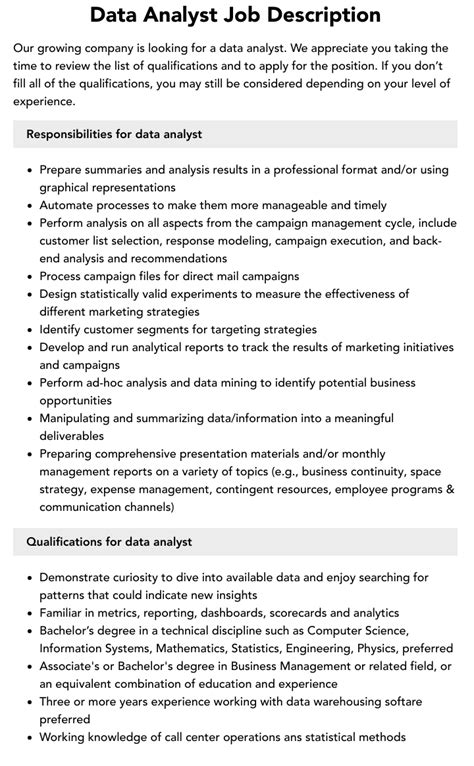
Big data has become an integral part of many industries, and companies are increasingly relying on data analysis to make informed decisions. As a result, the demand for big data analysts has grown sig...
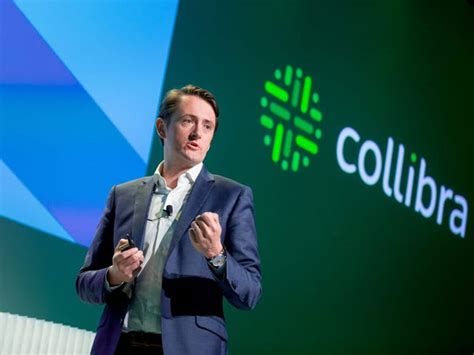
Big data has become an integral part of businesses across various industries. It refers to the massive volume of structured and unstructured data that organizations collect on a daily basis. This data...
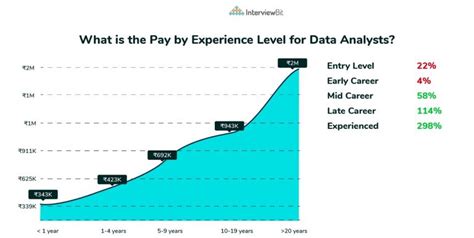
Big data has become an integral part of modern businesses, and the demand for skilled professionals who can analyze and interpret this vast amount of information is on the rise. One such profession th...
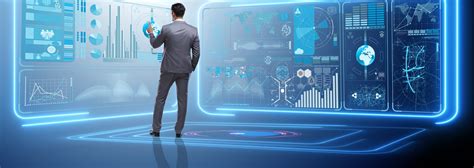
Big data has become an integral part of many industries, and the demand for skilled big data analysts is on the rise. These professionals are responsible for analyzing large sets of data to uncover pa...
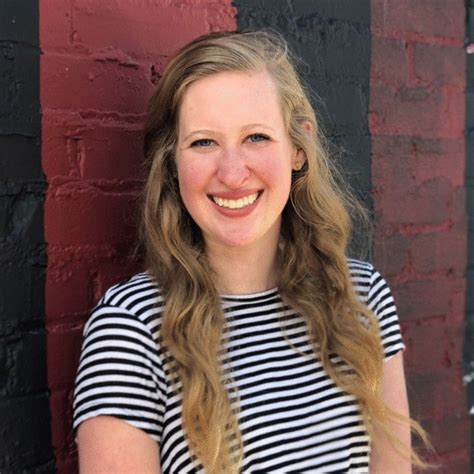
Southwest Airlines is one of the largest low-cost carriers in the world, serving millions of passengers each year. With a commitment to providing excellent customer service and affordable travel optio...
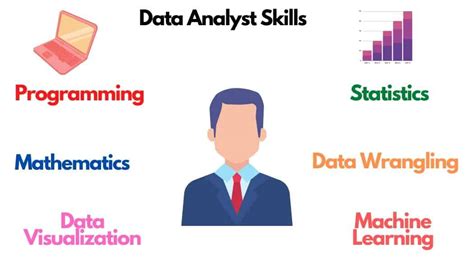
In today's digital age, data has become one of the most valuable assets for businesses. The ability to collect, analyze, and interpret large volumes of data has become crucial for making informed busi...
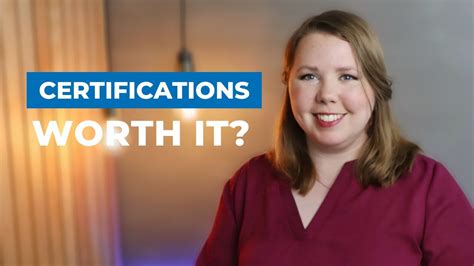
In today's digital age, data has become the new currency. Organizations across industries are collecting vast amounts of data, but the real value lies in the ability to analyze and derive insights fro...

Big data has become a buzzword in the business world, and organizations are increasingly relying on it to gain insights and make informed decisions. However, the sheer volume and complexity of big dat...
![Data Analyst Resume Samples & Templates [PDF+DOC] 2022 | Data Analyst Resumes Bot](/image/blogihisinfo/big-data-analyst-resume.jpg)
As the world becomes increasingly data-driven, the demand for skilled professionals who can analyze and interpret large volumes of data is on the rise. Big data analysts play a crucial role in helping...
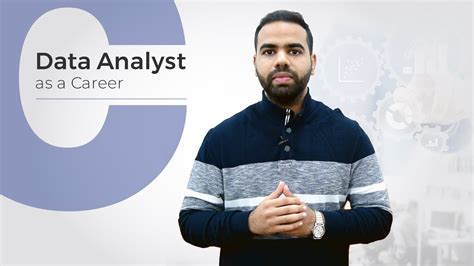
With the rapid growth of technology and the increasing importance of data in decision-making processes, the demand for skilled professionals in the field of big data analytics has skyrocketed. One suc...
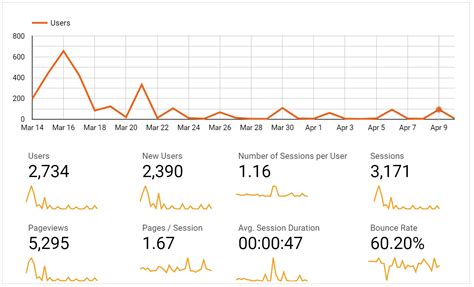
In today's digital age, businesses are constantly seeking ways to gain a competitive edge. One of the most effective strategies is leveraging digital marketing analytics to make data-driven decisions....
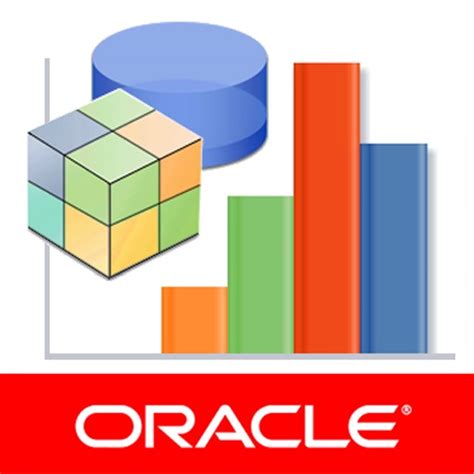
Oracle Business Intelligence (BI) is a powerful suite of tools and technologies designed to help organizations make data-driven decisions. By providing a comprehensive set of analytics, reporting, and...
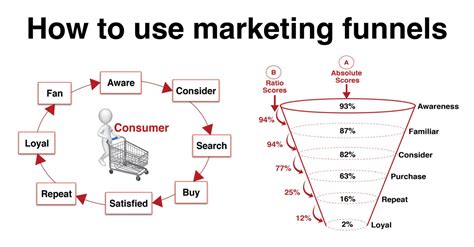
Marketing funnel optimization is a crucial aspect of any successful marketing strategy. It involves analyzing and improving each stage of the marketing funnel to maximize conversion rates and revenue....
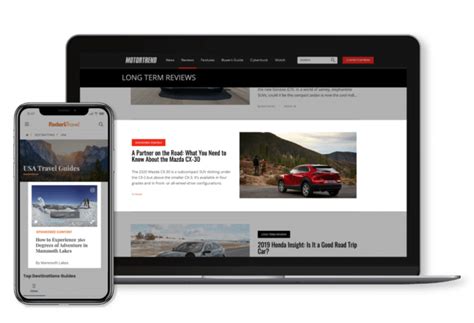
Native advertising has emerged as a powerful tool in the world of digital advertising. It seamlessly integrates promotional content with the user experience, making it less intrusive and more engaging...
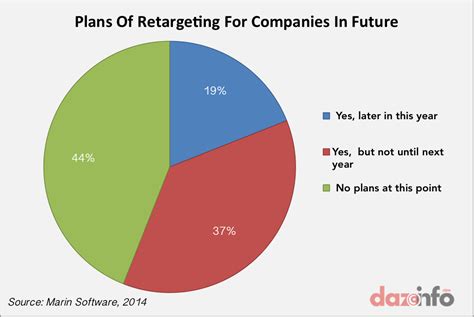
Retargeting advertising has become an essential strategy for businesses looking to maximize their online marketing efforts. It allows companies to reach out to potential customers who have already sho...
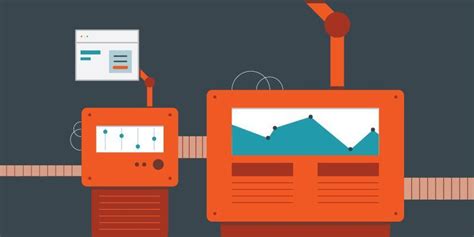
In today's digital age, businesses are constantly seeking innovative ways to streamline their marketing efforts and connect with customers more effectively. One such solution that has gained significa...
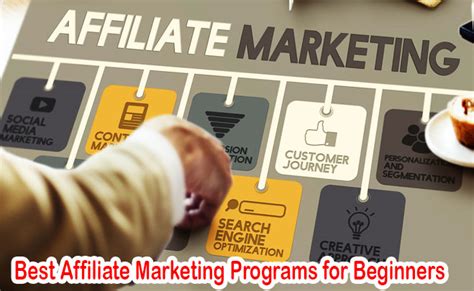
Affiliate marketing programs have gained immense popularity in recent years as a lucrative way for individuals and businesses to earn passive income. This comprehensive guide will provide you with an ...
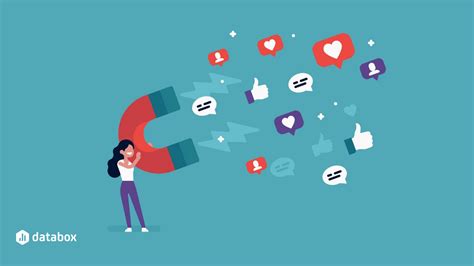
In today's digital age, influencer marketing has emerged as one of the most effective strategies for brand promotion. With the rise of social media platforms, influencers have gained significant popul...